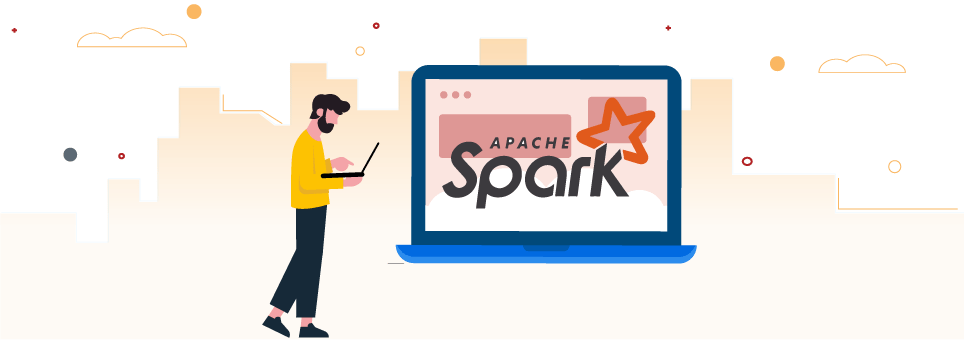
Apache Spark
Spark is based on the Hadoop distributed file system but does not use Hadoop MapReduce, but its own framework for parallel data processing, which starts with the insertion of data into persistent distributed data records (RDD) and distributed memory abstractions, which computes large Spark clusters in a way that fault-tolerant. Because data is stored in memory (and on disk if necessary), Apache Spark can be much faster and more flexible than the Hadoop MapReduce task for certain applications described below.
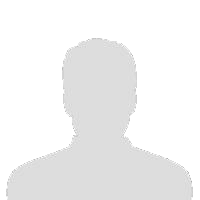
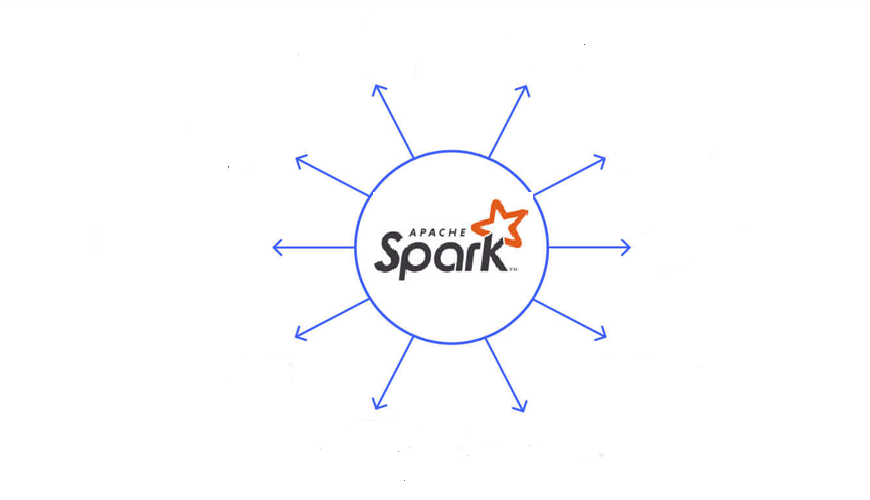
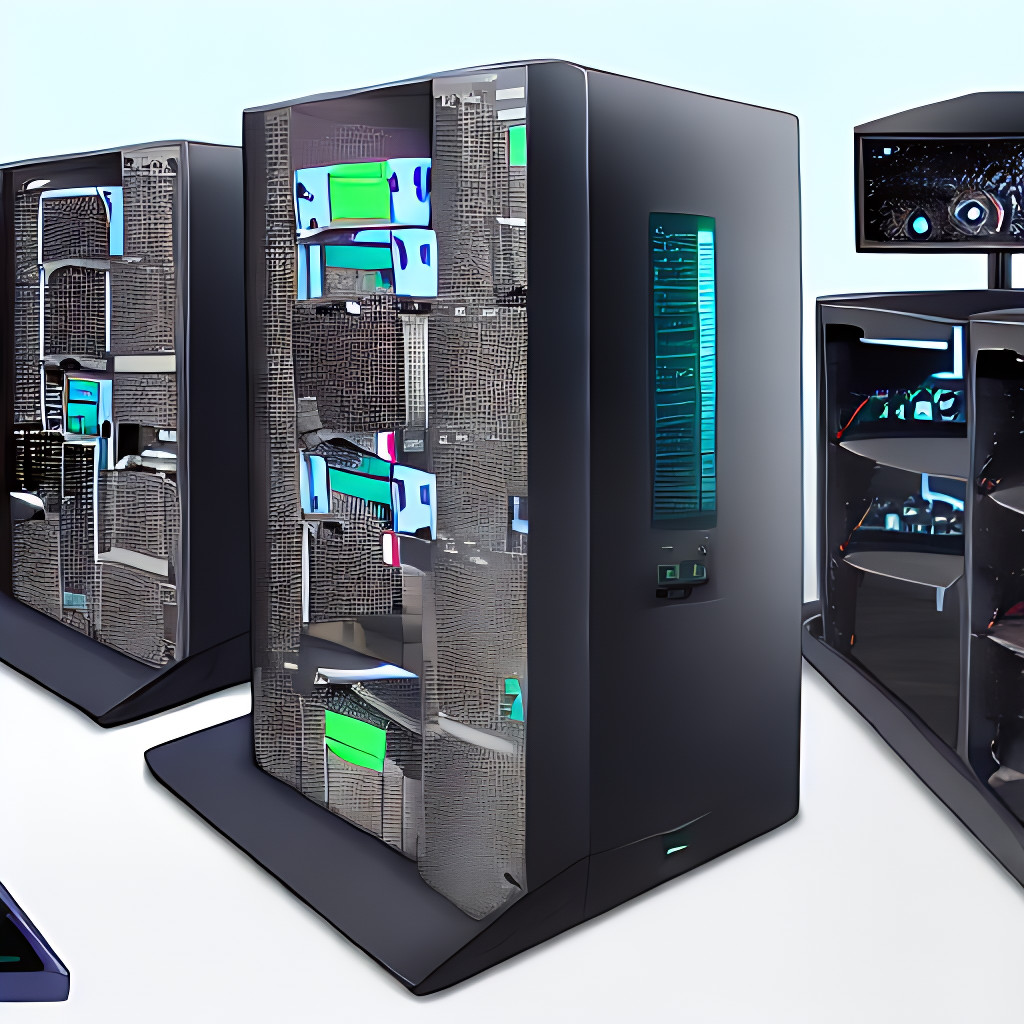
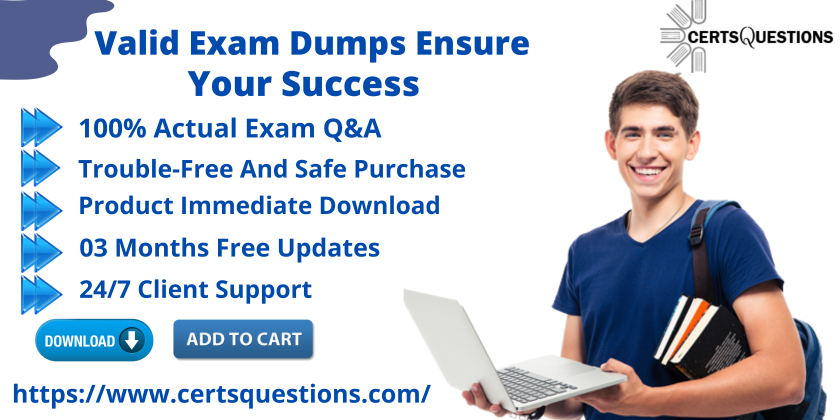
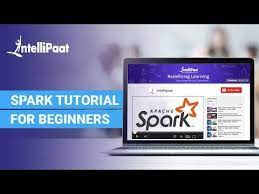
Proceeding with the targets to make Spark quicker, simpler, and more intelligent, Spark 2.4 broadens its degree with the accompanying highlights:A scheduler to help hindrance mode for better joining with MPI-based projects, for example distributed profound learning systemsPresent various inherent higher-request capacities to make it simpler to manage complex information types (i.e., cluster and guide)Offer trial help for Scala 2.12Permit the enthusiastic assessment of DataFrames in note pads for simple investigating and investigating.Present another inherent Avro information sourceNotwithstanding these new highlights, the delivery centers around usability, stability, and refinement, settling more than 1000 tickets.
Other remarkable highlights from Spark supporters include:Take out the 2 GB block size restriction [SPARK-24296, SPARK-24307]Pandas UDF enhancements [SPARK-22274, SPARK-22239, SPARK-24624]Picture composition information source [SPARK-22666]Flash SQL upgrades [SPARK-23803, SPARK-4502, SPARK-24035, SPARK-24596, SPARK-19355]Underlying record source enhancements [SPARK-23456, SPARK-24576, SPARK-25419, SPARK-23972, SPARK-19018, SPARK-24244]Kubernetes joining upgrade [SPARK-23984, SPARK-23146]In this blog entry, we momentarily sum up a portion of the greater level highlights and enhancements, and in the coming days, we will publish top to bottom sites for these highlights.
Flash additionally presents another mechanism of adaptation to non-critical failure for obstruction undertakings.
At the point when any boundary task fizzled in the center, Spark would cut short every one of the undertakings and restart the stage.Inherent Higher-request FunctionsBefore Spark 2.4, for controlling the unpredictable kinds (for example exhibit type) straightforwardly, there are two run of the mill arrangements: 1) detonating the settled design into singular lines, and applying a few capacities, and afterward making the construction once more.
The new underlying capacities can control complex sorts straightforwardly, and the higher-request capacities can control complex qualities with an unknown lambda work as you like, like UDFs yet with much better execution.You can peruse our blog on high-request capacities.So, you can learn Spark CertificationUnderlying Avro Data SourceApache Avro is a mainstream information serialization design.
Also, it gives:New capacities from_avro() and to_avro() to peruse and compose Avro information inside a DataFrame rather than simply documents.Avro consistent sorts support, including Decimal, Timestamp and Date type.
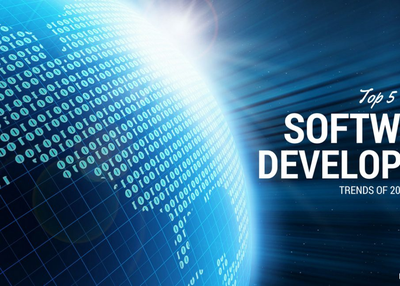
The move from the traditional desktop to the highly-versatile world of mobility is gaining in popularity.
Naturally, this decline does not necessarily mean that these two programming languages will be obsolete anytime soon.
Upon closer examination of these positions, you will see that those jobs lean more towards maintenance and support and less towards software development processes, such as programming.
#2 Cloud Computing is Gaining Popularity
It is commonplace now to see large corporations officially adopting the NoSQL policy in terms of their critical operations and opting for cloud computing processes, in terms of data placement.
In doing so, you can be rest assured that the tools, features, and services associated with your program are up-to-date and considered to be pertinent among your end users.
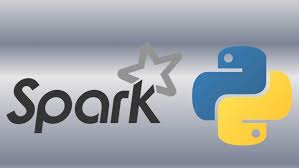
The “Global Apache Spark Market” report offers compound growth from the base year and projected until 2026.
The report is further fragmented on the basis of segmentation that involves product type, application, and geography.
Esticast Research and Consulting provides accurate market size and forecast in relation to the major five regions.
Apache Spark is a highly advanced, general, open source big data processing software, which has been designed to provide super-fast computation, especially for parallel processing programs.
The major advantage which is leading spark to replace Hadoop is that Apache Spark is nearly 100 times faster than Hadoop MapReduce owing to its advanced in-memory and parallel processing power.For Better Understanding, Try Sample PDF Brochure of Report (including full TOC, Tables and Figures) @ https://www.esticastresearch.com/report/apache-spark-market/#request-for-sampleMarket OverviewThe research report covers various developments across the geography of the Apache Spark market based on the tools of organic as well as inorganic growth strategies.
The market report presented provides key statistics based on the past and current status of the market coupled with key trends and opportunities.The report not only analyses factors responsible for impacting the Apache Spark market on the basis of the value chain but also evaluates industry forces that will highlight the market in the coming years.