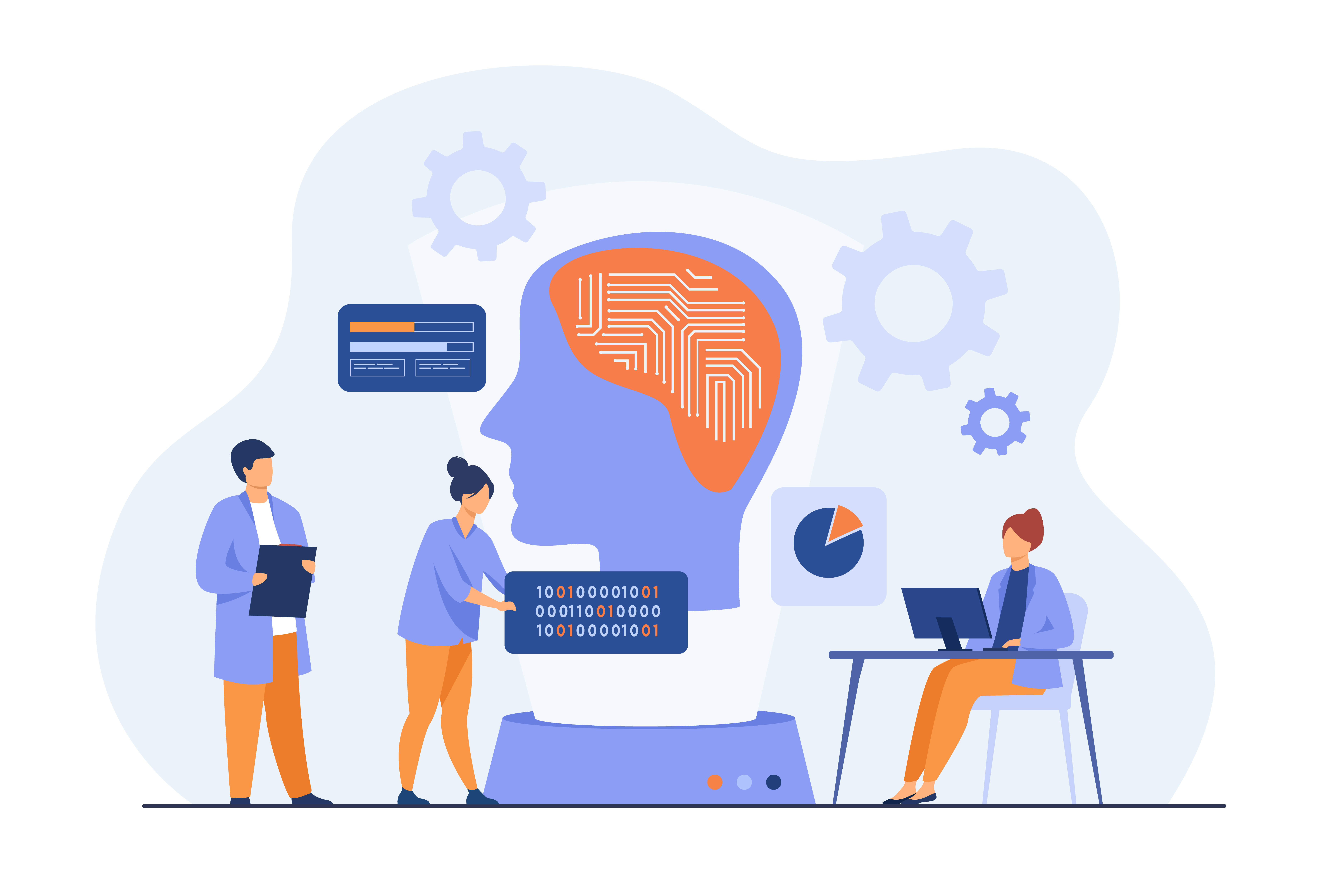
Table of Contents:
1. Introduction: The Evolving Landscape of Natural Language Processing
2. Sentiment Analysis: The Tip of the Iceberg in Text Analytics
3. Named Entity Recognition: Identification of Key Entities in Unstructured Text
4. Text Classification: Document Categorization Based on Content
5. Topic Modeling: Uncovering Hidden Themes and Patterns
6. Relationship Extraction: Identifying Relationships between Entities
7. Identifying Specific Information in Text: Question Answering
8. Text Summarization: Condensing Large Volumes of Text
9. Mastering Advanced NLP Techniques: Role of Data Science Courses for Working Professionals
10. Ethical Considerations in Applying NLP Techniques
11. Emerging Trends and Future Directions in NLP
12. Conclusion: Unlocking Full Potential of Text Data using Advanced NLP
Introduction: Natural Language Processing Evolving to Full Potential
The ability to extract meaningful insights from the most common form of data available in organizations today, unstructured text data, has been an important skill for a data scientist or business analyst in this age of big data. Observing this trend, Natural Language Processing, a subfield of artificial intelligence, has also evolved to become a highly potent tool for the analysis and understanding of human languages, thus letting organizations unlock the hidden value within their textual data.
While sentiment analysis used to be the generic technique for the extraction of insights from text, the development of NLP has been immense and offers a wide plethora of advanced techniques that go beyond simple sentiment classification. In this paper, we will have a look at these state-of-the-art NLP techniques, their applications, and the need for data science courses among professionals to master such skills.
Sentiment Analysis: Tip of the Text Analytics Iceberg
Sentiment analysis has been one of the most widespread applications of NLP in the last couple of decades. It determines the sentiment or emotional tone conveyed by a piece of text. Classifying the text as positive, negative, or neutral gives insight into customer opinion, brand perception, and market trends.
Sentiment Analysis represents, however, only the tip of the iceberg when it comes to the real possibilities of NLP. And with further development in this field, data scientists and NLP practitioners have developed a bunch of much more sophisticated techniques that can unlock even deeper insights from text data, way beyond simple sentiment classification.
Named Entity Recognition: Identifying Key Entities in Unstructured Text
One of the NLP techniques for identifying and extracting named entities, such as people, organizations, locations, and dates, from unstructured text is named entity recognition. This function helps organizations comprehend their text data better by detecting these key entities and, hence, the context and relationships within, allowing targeted analysis and decision-making.
The NER applications lie in customer relationship management, risk analysis, and content recommendation. The course on these topics is covered separately in detail in any of the experienced professional courses in Data Science, which also includes hands-on experience in implementing and applying these techniques to real-world text data.
Text Classification: Document Categorization by Content
It means classifying text into predefined categories or labels based on its content. This technique may be applied to a number of varied tasks like topic recognition, sentiment analysis, and spam detection.
The text classification models can then become critiquing in nature for the proper classification of the text data—in fact, in cases that are complicated or otherwise open to multiple interpretations. This means that modules on text classification find a place in professional courses on data science, thereby arming such learners with the capability to develop and deploy such models within their respective organizations.
Topic Modeling: Uncovering Hidden Themes and Patterns
It is unsupervised; hence, topic modeling automatically generates the themes or topics underlying a large collection of text documents. Analyzing co-occurrences of words and phrases, topic modeling algorithms discover hidden semantic structures in data to gain insight into the principal themes or concepts being discussed.
Such applications of topic modeling include content recommendation, market research, and social media analysis. This usually is the case for courses in data science that are targeted at experienced professionals, as they are supposed to know how to implement and understand such models using standard NLP libraries and frameworks.
Relationship Extraction: Relations Between Entities
It is the process of detecting and extracting relations between named entities in the text. This can be further extended to discover complex networks of relationships like relationships between people, organizations and events.
Applications in relationship extraction range from fraud detection to social network analysis, knowledge graph construction, and many more. As such, most data science training for experienced professionals broadens out to modules on relationship extraction, with a focus on equipping the learner to develop and apply the techniques to their own textual data.
Question Answering: Extracting Specific Information from Text
QA is an NLP technique that tries to return a factually correct and concise answer based on the text. Extracting relevant information and generating answers to various questions, QA systems develop advanced understanding and reasoning capabilities over languages.
QA finds applications in customer service, knowledge management, and delivery of educational content. State-of-the-art QA is often taught in data science courses for experienced professionals through example-based training in building and deploying such systems using state-of-the-art NLP models and architectures.
Text Summarization: Condensing Large Volumes of Text
Text summarization is the process of generating a Macrocalled summary of the longer text that captures the main ideas and general points, omitting unnecessary length. This technique thus becomes very important in distilling insights from vast amounts of text data, be it news articles, research papers, or customer reviews.
Most data science courses for Working professionals would include text summarization modules that cover both extractive and abstractive approaches to this task. The students learn to implement and evaluate text summarization models and how to apply the techniques worked out to business problems in the real world.
How Data Science Courses for Experienced Professionals Help Master Advanced NLP
Advanced NLP courses are also very instrumental to seasoned professionals aiming to broaden their skill areas in data science. They provide an in-depth course outline, from theoretical underpinning with reference to NLP to practical experience regarding implementation and application using state-of-the-art deep learning frameworks and libraries.
It fuses lectures, tutorials, and project-based learning to achieve a whole learning environment wherein students of data science courses are exposed to cutting-edge NLP methods and their applications. Students of the program get exposure to modern research papers, case studies from the industry, and mentoring by experts, which imbues them with the required confidence and expertise to solve real challenges and be innovative in the field of text analytics.
Ethical Considerations: Applications of NLP Techniques
Innovative applications of NLP shall increasingly require an eye to the ethical ramifications of techniques. Issues on bias, privacy, transparency, and others have to be clearly accounted for in such a way that insights and decisions realized from an NLP model do not perpetuate or amplify the inequities already in society.
Courses on data science targeted at professionals are entering into having modules on the ethics of NLP. These would cover concerns such as algorithmic bias, privacy in data, and requirements on transparency for models of machine learning. It is through this type of education in the responsible application of advanced NLP techniques that the culture of ethical data science promotes a responsible and socially aware implementation of NLP.
Emerging Trends and Future Directions in NLP
The domain of NLP keeps evolving with new techniques, architectures, and applications that crop up once in a while. From the rise of the transformer-based models to the integration of NLP with other AI paradigms, the future of NLP opens up a vista of exciting opportunities and complex challenges.
Guests and panels of leading researchers and industry experts teach most of the emerging trends and innovations in data science courses to experienced professionals. Students can, with dynamic knowledge, place themselves at the very top in this fast-evolving landscape, help shape progress in NLP technologies, and applications.
Conclusion: Unlocking Full Potential of Text Data with Advanced NLP
The most central competence that organizations seem to require in the big data age is extracting meaningful insights from unstructured text data. While sentiment analysis was hitherto the go-to technique for text analytics, what began with sentiment analysis currently represents a field way beyond this simple method of advanced techniques that can unleash deeper insights and drive more informed decision-making in the field of NLP.
Advanced NLP methods professional data science courses are designed to enable aspiring data scientists and business analysts to develop an appropriate body of knowledge, skillset, and ethical groundwork so that they can apply these methods to solve real-world problems. It imparts theoretical instruction, hands-on experiences, and exposure to the state-of-the-art research and industry practices in a nutshell to be equipped with tools and a mindset to succeed in this fast-evolving field.
Effective usage of advanced NLP techniques will allow one to leverage the future of data science and AI. Professionals who are in command of such powerful tools and techniques would open new frontiers of text analytics and insights within their domain, driving progress and innovation toward the shaping of the future data-driven world.