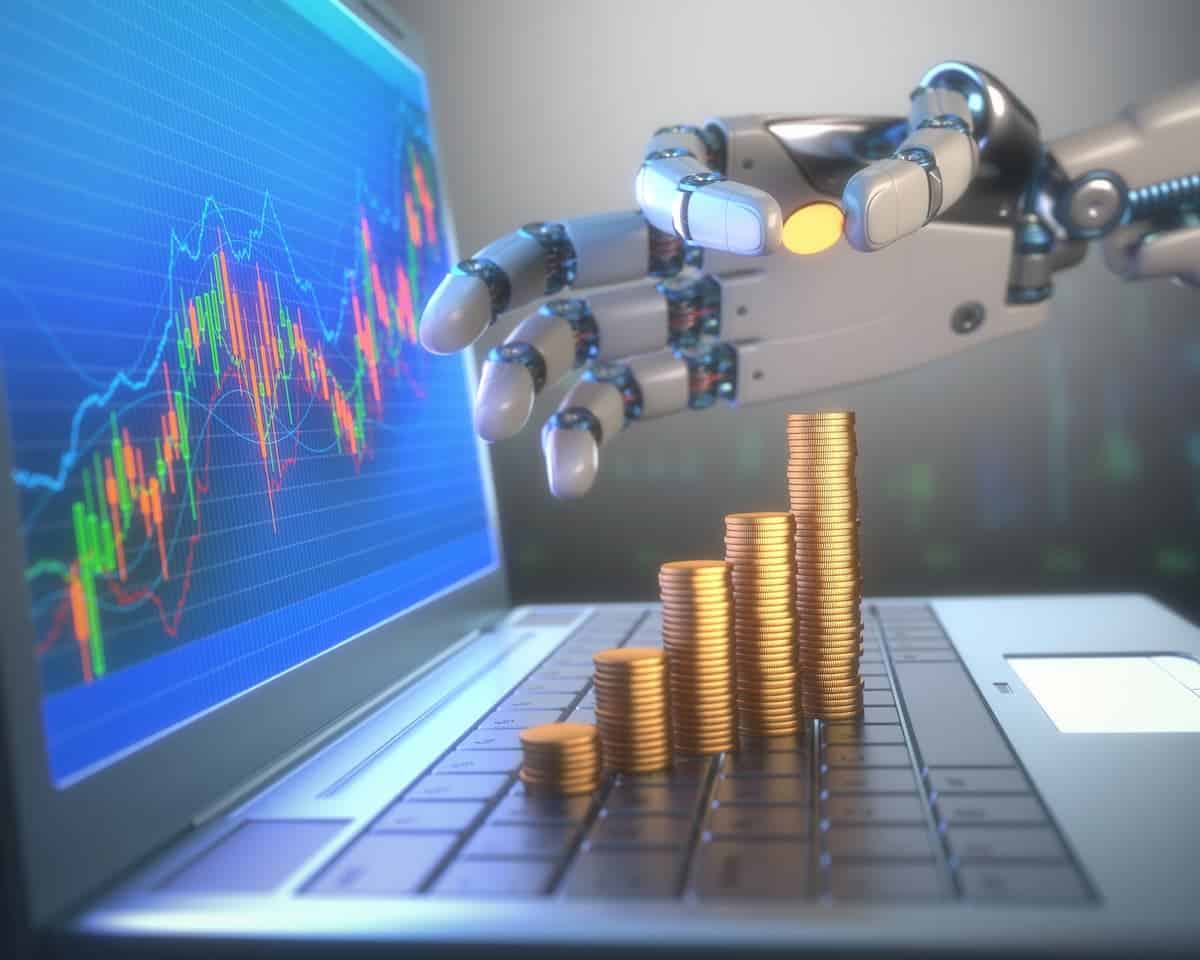
In the world of finance, quantitative methods serve as the
backbone for decision-making, risk management, and the pricing of financial instruments. One of the most prominent areas within quantitative finance is derivatives pricing. Derivatives, financial contracts that derive their value from an underlying asset, are fundamental tools for hedging, speculation, and risk transfer. In this blog, we will explore the techniques used in derivatives pricing and their wide-ranging applications in finance.
Understanding Derivatives
Derivatives are financial contracts whose value depends on
the performance of an underlying entity such as stocks, bonds, commodities, currencies, or market indices. Common types of derivatives include:
Options: Contracts that give the buyer the right, but not the obligation, to buy or sell an asset at a predetermined price before a specific date.
Futures and Forwards: Agreements to buy or sell an asset at a predetermined price on a future date.
Swaps: Contracts where two parties exchange cash flows or financial instruments, such as interest rate swaps.
Credit Derivatives: Instruments used to manage credit risk, such as credit default swaps (CDS).
The pricing of these instruments is crucial for their effective use in financial markets. Mispricing can lead to significant risks and financial losses.
Techniques in Derivative Pricing
1. Arbitrage-Free Pricing
The concept of arbitrage—risk-free profit opportunities—is fundamental to derivatives pricing. Arbitrage-free pricing assumes that markets are efficient and that there are no opportunities to make a profit without taking on risk. This principle underpins models such as the Black-Scholes model.
2. The Black-Scholes Model
Developed by Fischer Black, Myron Scholes, and Robert Merton, the Black-Scholes model is one of the most widely used tools for pricing options. The model is based on several assumptions, including constant volatility, no dividends, and the ability to hedge perfectly. The formula for a European call option is given by:
Where:
Current stock price
Strike price
Risk-free interest rate
Volatility
Time to maturity
Cumulative distribution function of the standard normal distribution
3. Monte Carlo Simulations
Monte Carlo methods are computational techniques used to model and price complex derivatives by simulating thousands or even millions of random price paths of the underlying asset. These methods are especially useful for pricing path-dependent options, such as Asian options or barrier options, which depend on the asset’s price trajectory rather than its final value.
4. Finite Difference Methods
These numerical techniques solve partial differential equations (PDEs) that arise in derivatives pricing models. For instance, the Black-Scholes PDE can be solved using finite difference methods to obtain the price of an option. Common approaches include:
Explicit methods
Implicit methods
Crank-Nicolson methods
5. Binomial and Trinomial Trees
Tree-based models are intuitive and flexible techniques for pricing options. The binomial tree model divides the time to expiration into discrete intervals and models the price of the underlying asset as moving up or down by a specific factor at each step. Trinomial trees add state, allowing the price to remain constant during a step.
6. Stochastic Differential Equations (SDEs)
SDEs are used to model the dynamic behavior of asset prices. These equations incorporate randomness and are solved using advanced mathematical tools such as Ito’s Lemma. The Black-Scholes model itself is derived from an SDE that describes the evolution of asset prices under geometric Brownian motion.
7. Machine Learning and AI
With advancements in computational power, machine learning techniques have begun to play a role in derivatives pricing. Neural networks, for example, can approximate complex pricing functions and are particularly useful when traditional models fail to capture market nuances.
Applications of Derivatives Pricing
1. Risk Management
Derivatives are essential tools for managing financial risk. For example, a company can use options to hedge against adverse movements in currency exchange rates or commodity prices. Accurate pricing ensures that the cost of such hedging strategies is fair and transparent.
2. Portfolio Management
In portfolio management, derivatives are used to enhance returns or mitigate risks. For instance, a portfolio manager might use futures contracts to adjust the exposure of a portfolio to a particular asset class without buying or selling the underlying assets.
3. Structured Products
Structured products are custom financial instruments created using derivatives. These products are tailored to meet specific investor needs, such as capital protection or enhanced income. Pricing these instruments accurately requires a deep understanding of derivatives pricing techniques.
4. Market Making
Market makers provide liquidity by quoting bid and ask prices for derivatives. To do so profitably, they rely on robust pricing models to ensure that their quoted prices reflect the true value of the instruments while accounting for transaction costs and risks.
5. Regulatory Compliance
Regulators often require financial institutions to mark derivatives to market—that is, to value them at their current market price. Accurate pricing models are critical for meeting these requirements and ensuring financial stability.
6. Credit Risk Management
Credit derivatives, such as credit default swaps, are used to manage credit risk. Pricing these instruments involves modeling the likelihood of default and the recovery rate, both of which require sophisticated quantitative techniques.
7. Exotic Derivatives
Exotic derivatives, such as rainbow options or quanto options, have features that make them more complex than standard derivatives. Pricing these instruments requires advanced techniques like Monte Carlo simulations and numerical methods.
Challenges in Derivatives Pricing
Despite significant advancements, derivatives pricing is not without its challenges:
Market Assumptions: Models like Black-Scholes rely on assumptions such as constant volatility and no transaction costs, which are often unrealistic.
Calibration: Models must be calibrated to market data, which can be challenging due to data noise and changing market conditions.
Computational Complexity: Advanced models and numerical techniques can be computationally intensive, especially for high-dimensional problems.
Black Swan Events: Unforeseen events can lead to extreme market movements that traditional models fail to predict.
The Future of Derivatives Pricing
The future of derivatives pricing lies at the intersection of mathematics, data science, and technology. Emerging trends include:
Machine Learning Integration: AI models that can adapt to changing market conditions and improve prediction accuracy.
Quantum Computing: Leveraging quantum algorithms to solve complex pricing problems more efficiently.
Alternative Data Sources: Using non-traditional data, such as social media sentiment, to enhance pricing models.
Sustainable Finance: Pricing derivatives linked to ESG (Environmental, Social, and Governance) metrics.
Conclusion
Quantitative finance and derivatives pricing represent the confluence of mathematics, statistics, and computational science. These techniques are essential for modern financial markets, enabling participants to manage risk, allocate resources efficiently, and innovate with new financial products. While challenges persist, ongoing advancements in technology and methodology promise to make derivatives pricing more accurate, robust, and accessible. For anyone aspiring to enter this dynamic field, mastering these techniques is not just a requirement but a gateway to shaping the future of finance.